Dr. Josué Aarón López-Leyva, Professor and Researcher at the School of Engineering at CETYS University International Campus in Ensenada, has delved into red and white wine quality analysis using advanced Machine-Learning algorithms.
Machine-Learning, a form of Artificial Intelligence, allows computer systems to learn from collected data instead of relying on explicit programming, providing valuable insights for decision-making processes.
Dr. López’s project aims to assess more than ten physicochemical parameters present in various red and white wine samples and establish correlations between each parameter, wine type, and its perceived quality determined by sensory analysis. Sensory analysis, in this context, involves describing wines based on their organoleptic properties unique to each variety.
The quantitative findings of the project reveal that the quality of red and white wines is most closely associated with two physicochemical parameters: alcohol percentage and the quantity of volatile acids. Other parameters hold more relevance in red wines than in white wines and vice versa, such as citric acid and density.
However, despite the project’s sophisticated Machine-Learning approach and the analysis of diverse physicochemical parameters, it was found that determining wine quality with utmost precision remains a challenging task, achieving an accuracy of approximately 70%.
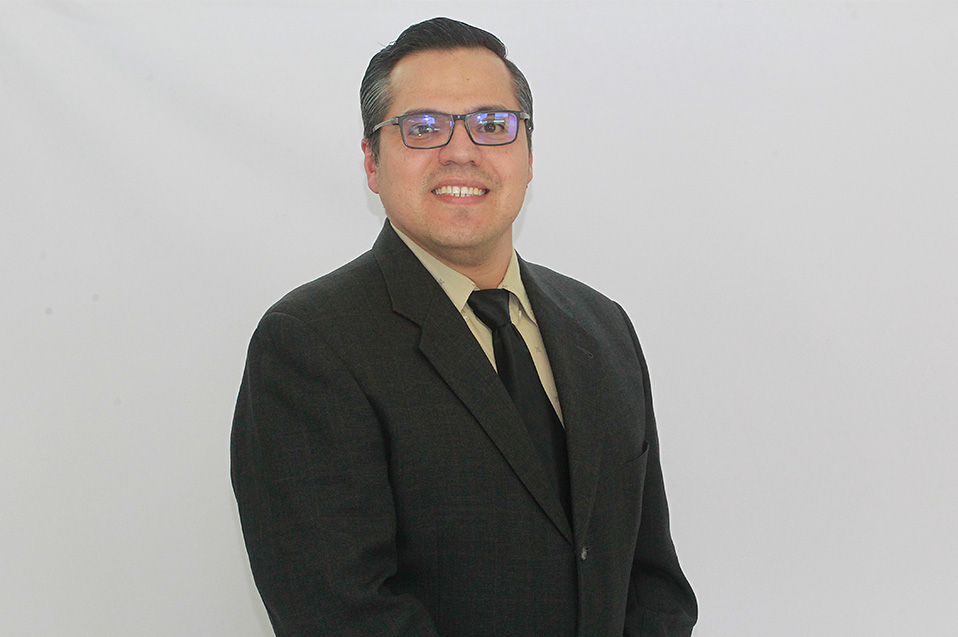
«The implications of these findings are diverse. First, the end consumer of wine is hypothetically responsible for the remaining 30% of accuracy needed to correctly classify wine quality. In other words, each taster assigns a quality level based on aspects such as color, lightness, consistency, effervescence, intensity, complexity, alcohol content, acidity, etc. – aspects related to visual inspection, olfactory examination, and taste testing. Second, it might be necessary to consider more physicochemical parameters and/or increase the number of red and white wine samples across all quality levels, ensuring they are not unintentionally skewed towards average-quality wines. Currently, we observe that 78.5% of the samples of red and white wines were classified by tasters as medium quality. This could introduce a potential bias in the analysis of lower and higher quality wines,” explains the researcher.
Furthermore, Dr. López highlighted that the Machine-Learning algorithms’ performance showed that medium-quality wines -red and white- demonstrated the highest accuracy (73%) in determining their quality based on the physicochemical parameters. Conversely, lower and higher-quality wines experienced significantly lower quality prediction accuracy, with 10% and 17%, respectively. Nonetheless, despite these challenges, the Machine-Learning results can provide valuable insights into monitoring physicochemical parameters to control the final quality of the wine. However, it is essential to acknowledge the vital role of customers and tasters in the ultimate decision-making process, as wine quality determination remains a multifaceted and subjective affair.
To conclude, Dr. López emphasized the immense potential of applying Machine-Learning across various stages of the wine production process, encompassing formal and factual sciences. Encouraging further research in Machine-Learning, particularly concerning administrative, engineering, social, and environmental aspects related to the wine industry, could enable a deeper understanding of the complex factors influencing wine quality. In addition, such research projects offer the opportunity to compare the quality and physicochemical parameters of wines from diverse national and international regions, climates, and grape varieties, ultimately contributing to the advancement of the wine industry.
Leave your comment